Doing Academic Research for Industry Impact.
The Media Neuroscience Lab remains committed to the mission of advancing research-based solutions that contribute towards diverse, equitable, and inclusive practices in storytelling. MNL utilizes its extensively validated, hybrid content analysis pipeline combining human and artificial intelligence to assess diversity, equity, and inclusion (DEI) metrics across textual and audiovisual content.
Our computational, large-scale analyses are enriched and supplemented by manual, human-expert codings to (a) ensure accuracy of automatically derived DEI metrics, (b) fine-tune artificial content classification systems, and (c) develop and train novel algorithms for extracting DEI from narratives.
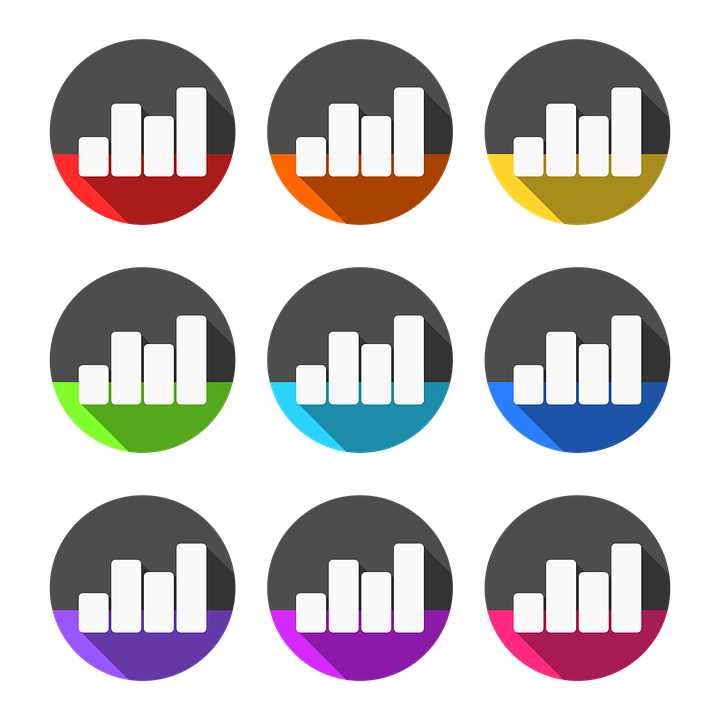
Finding Character Diversity in International Cinema.
In the international film industry, gender, racial, and sexual minorities remain underrepresented. Characters from these groups receive less screen time, fewer central story positions, and frequently inherit plotlines, motivations, and actions that are primarily driven by characters belonging to dominant demographic groups.
Our research combines state-of-the-art methodological tools from computer vision and network science to develop a content analytic framework for providing clearly defined, standardized, and scalable DEI metrics. We take stock of minorities’ structural positions, cinematographic representations, and emotional portrayals across the largest repository of international cinematic content ever compiled for inclusion research in film productions.
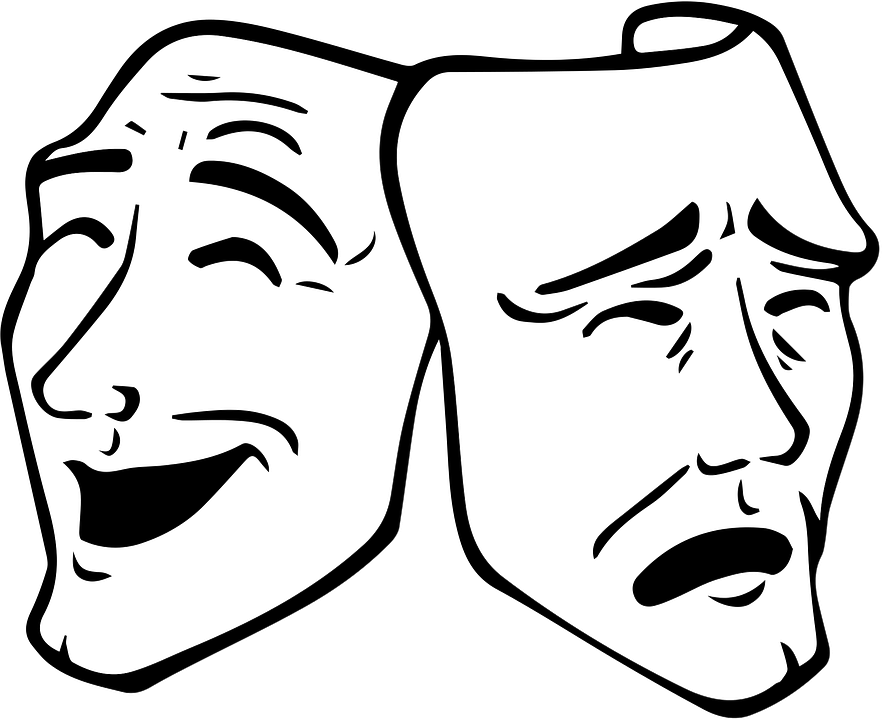
Ensuring Individual Equity in Global News.
In light of the recent protests against police brutality towards minority groups, and their important implications for politicized and racialized discourse in popular culture, our research focuses on the representation of racial minorities in media news coverage across popular global news stations. We extend research related to on-air racial- representation in news coverage by leveraging the unique positions of cable and video-streaming platforms as broadcasting mediums.
We further propose computational approaches for the detection and identification of the race of broadcasters, commentators, and speakers in news clips from mainstream global news channels via text classification and computer vision techniques and investigate how the on-air racial representation of broadcasters, commentators, and speakers interacts with news popularity and news sentiment measures.
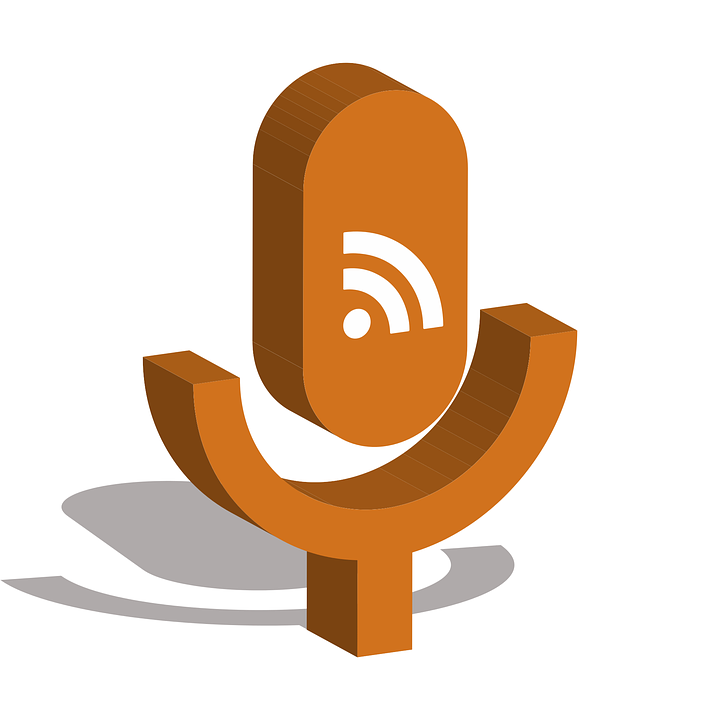
Building Human Inclusion in Social Media.
In the current cultural climate, it is imperative for media organizations to understand whether the content they deliver to users represents their audiences. In the case of social media companies this is particularly important as popular social media platforms are used by millions of daily active users globally. This means that social media content today reaches a audiences that is more diverse across race/ethnicity, gender, and sexuality than previous generations.
Pairing our computational methods with traditional human assessment techniques has resulted in the provisioning of a scalable procedure to reliably evaluate a large quantity of social media content in a relatively short amount of time. The outcome is a deeper understanding of how social media content reflects its audiences with regard to gender, race/ethnicity, and other inclusion metrics.
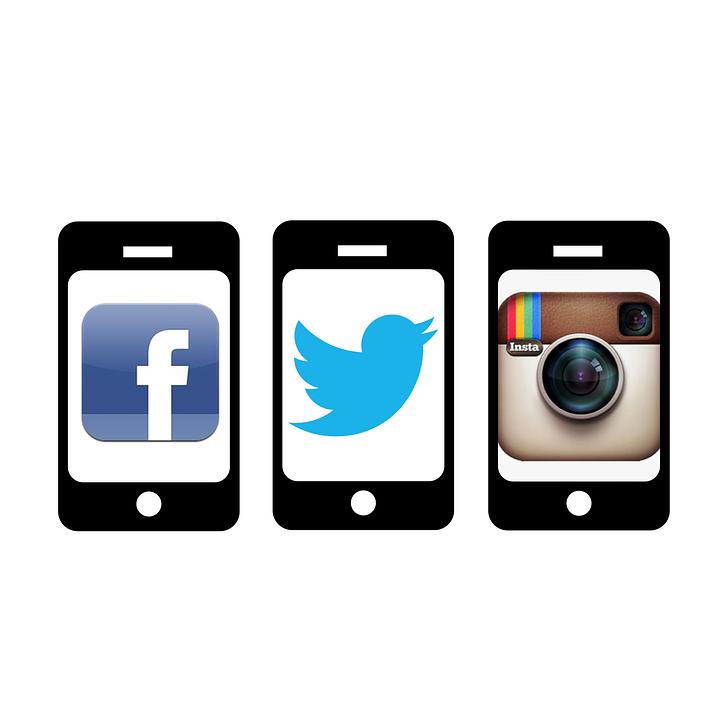
Our Partners
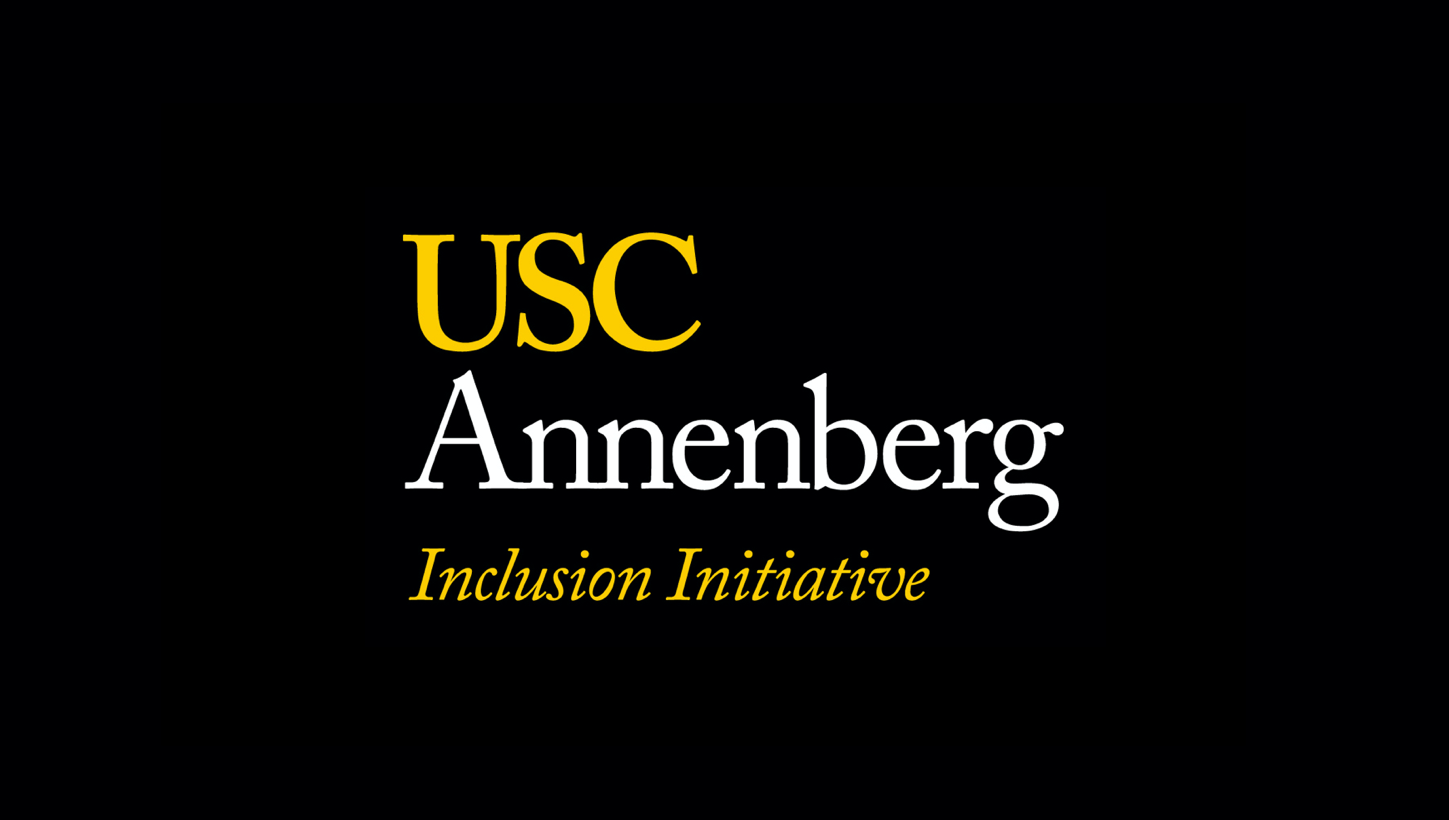
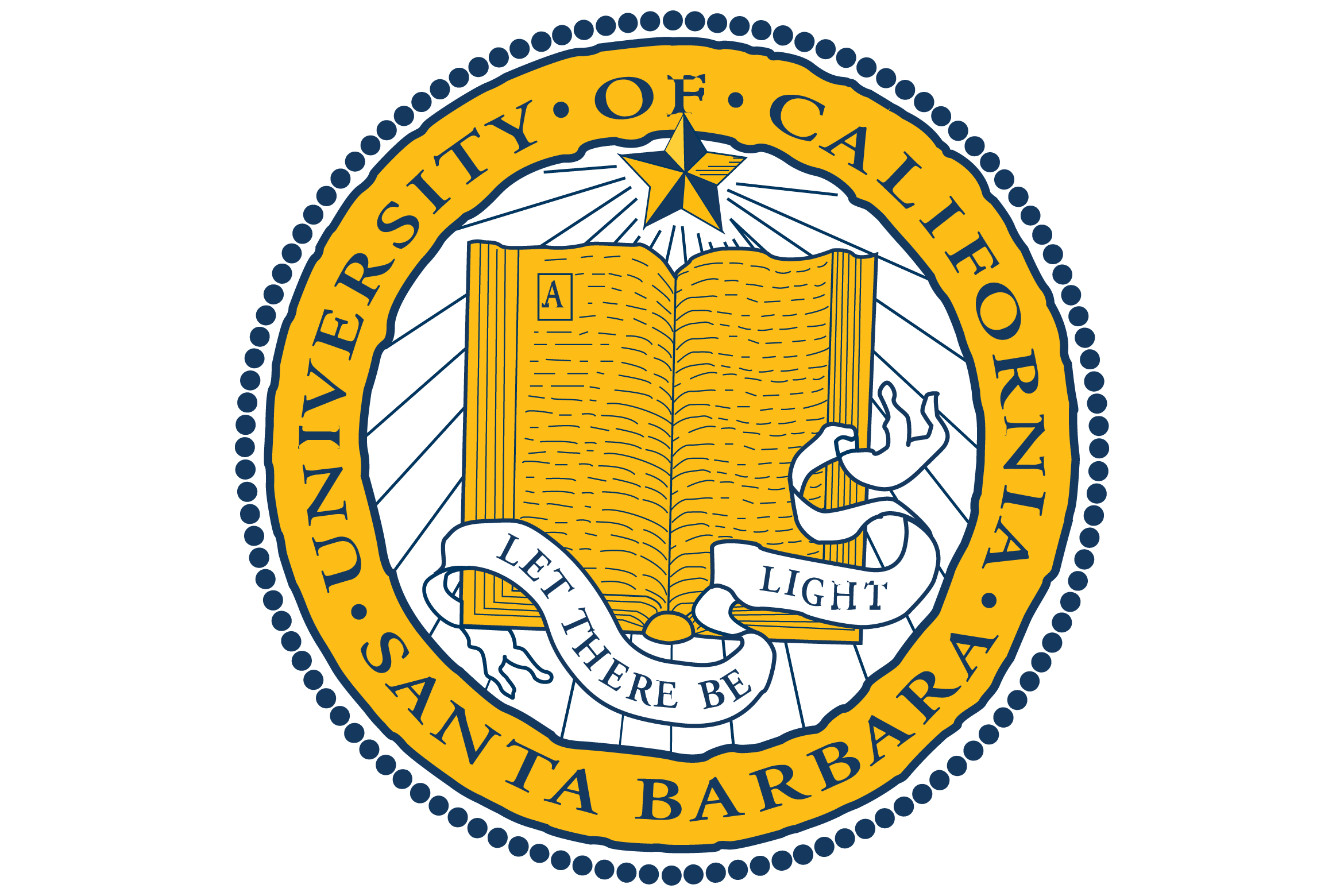